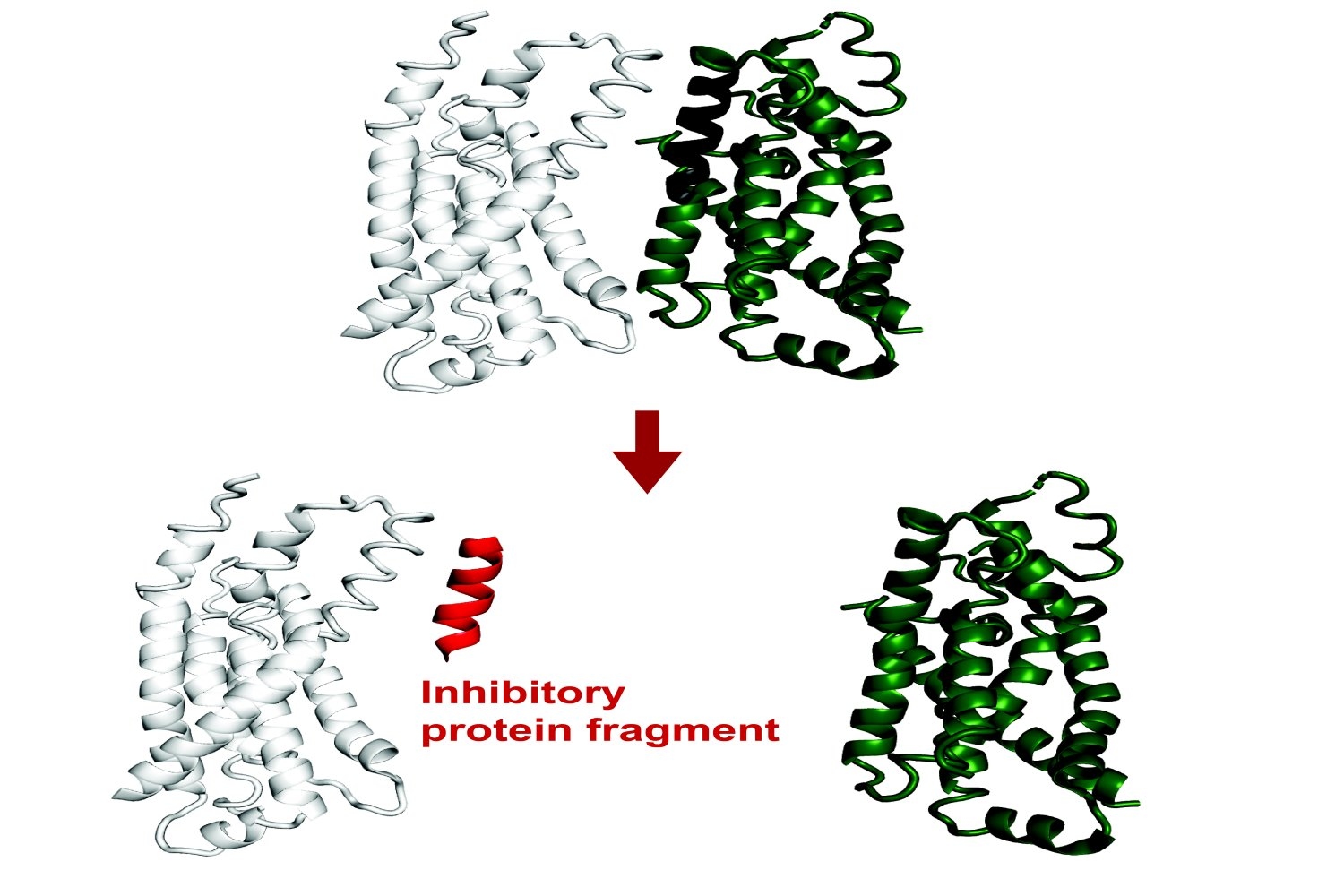
All biological function is dependent on how different proteins interact with each other. Protein-protein interactions facilitate everything from transcribing DNA and controlling cell division to higher-level functions in complex organisms.
Much remains unclear, however, about how these functions are orchestrated on the molecular level, and how proteins interact with each other — either with other proteins or with copies of themselves.
Recent findings have revealed that small protein fragments have a lot of functional potential. Even though they are incomplete pieces, short stretches of amino acids can still bind to interfaces of a target protein, recapitulating native interactions. Through this process, they can alter that protein’s function or disrupt its interactions with other proteins.
Protein fragments could therefore empower both basic research on protein interactions and cellular processes, and could potentially have therapeutic applications.
Recently published in Proceedings of the National Academy of Sciences, a new method developed in the Department of Biology builds on existing artificial intelligence models to computationally predict protein fragments that can bind to and inhibit full-length proteins in E. coli. Theoretically, this tool could lead to genetically encodable inhibitors against any protein.
The work was done in the lab of associate professor of biology and Howard Hughes Medical Institute investigator Gene-Wei Li in collaboration with the lab of Jay A. Stein (1968) Professor of Biology, professor of biological engineering, and department head Amy Keating.
Leveraging machine learning
The program, called FragFold, leverages AlphaFold, an AI model that has led to phenomenal advancements in biology in recent years due to its ability to predict protein folding and protein interactions.
The goal of the project was to predict fragment inhibitors, which is a novel application of AlphaFold. The researchers on this project confirmed experimentally that more than half of FragFold’s predictions for binding or inhibition were accurate, even when researchers had no previous structural data on the mechanisms of those interactions.
“Our results suggest that this is a generalizable approach to find binding modes that are likely to inhibit protein function, including for novel protein targets, and you can use these predictions as a starting point for further experiments,” says co-first and corresponding author Andrew Savinov, a postdoc in the Li Lab. “We can really apply this to proteins without known functions, without known interactions, without even known structures, and we can put some credence in these models we’re developing.”
One example is FtsZ, a protein that is key for cell division. It is well-studied but contains a region that is intrinsically disordered and, therefore, especially challenging to study. Disordered proteins are dynamic, and their functional interactions are very likely fleeting — occurring so briefly that current structural biology tools can’t capture a single structure or interaction.
The researchers leveraged FragFold to explore the activity of fragments of FtsZ, including fragments of the intrinsically disordered region, to identify several new binding interactions with various proteins. This leap in understanding confirms and expands upon previous experiments measuring FtsZ’s biological activity.
This progress is significant in part because it was made without solving the disordered region’s structure, and because it exhibits the potential power of FragFold.
“This is one example of how AlphaFold is fundamentally changing how we can study molecular and cell biology,” Keating says. “Creative applications of AI methods, such as our work on FragFold, open up unexpected capabilities and new research directions.”
Inhibition, and beyond
The researchers accomplished these predictions by computationally fragmenting each protein and then modeling how those fragments would bind to interaction partners they thought were relevant.
They compared the maps of predicted binding across the entire sequence to the effects of those same fragments in living cells, determined using high-throughput experimental measurements in which millions of cells each produce one type of protein fragment.
AlphaFold uses co-evolutionary information to predict folding, and typically evaluates the evolutionary history of proteins using something called multiple sequence alignments for every single prediction run. The MSAs are critical, but are a bottleneck for large-scale predictions — they can take a prohibitive amount of time and computational power.
For FragFold, the researchers instead pre-calculated the MSA for a full-length protein once, and used that result to guide the predictions for each fragment of that full-length protein.
Savinov, together with Keating Lab alumnus Sebastian Swanson PhD ’23, predicted inhibitory fragments of a diverse set of proteins in addition to FtsZ. Among the interactions they explored was a complex between lipopolysaccharide transport proteins LptF and LptG. A protein fragment of LptG inhibited this interaction, presumably disrupting the delivery of lipopolysaccharide, which is a crucial component of the E. coli outer cell membrane essential for cellular fitness.
“The big surprise was that we can predict binding with such high accuracy and, in fact, often predict binding that corresponds to inhibition,” Savinov says. “For every protein we’ve looked at, we’ve been able to find inhibitors.”
The researchers initially focused on protein fragments as inhibitors because whether a fragment could block an essential function in cells is a relatively simple outcome to measure systematically. Looking forward, Savinov is also interested in exploring fragment function outside inhibition, such as fragments that can stabilize the protein they bind to, enhance or alter its function, or trigger protein degradation.
Design, in principle
This research is a starting point for developing a systemic understanding of cellular design principles, and what elements deep-learning models may be drawing on to make accurate predictions.
“There’s a broader, further-reaching goal that we’re building towards,” Savinov says. “Now that we can predict them, can we use the data we have from predictions and experiments to pull out the salient features to figure out what AlphaFold has actually learned about what makes a good inhibitor?”
Savinov and collaborators also delved further into how protein fragments bind, exploring other protein interactions and mutating specific residues to see how those interactions change how the fragment interacts with its target.
Experimentally examining the behavior of thousands of mutated fragments within cells, an approach known as deep mutational scanning, revealed key amino acids that are responsible for inhibition. In some cases, the mutated fragments were even more potent inhibitors than their natural, full-length sequences.
“Unlike previous methods, we are not limited to identifying fragments in experimental structural data,” says Swanson. “The core strength of this work is the interplay between high-throughput experimental inhibition data and the predicted structural models: the experimental data guides us towards the fragments that are particularly interesting, while the structural models predicted by FragFold provide a specific, testable hypothesis for how the fragments function on a molecular level.”
Savinov is excited about the future of this approach and its myriad applications.
“By creating compact, genetically encodable binders, FragFold opens a wide range of possibilities to manipulate protein function,” Li agrees. “We can imagine delivering functionalized fragments that can modify native proteins, change their subcellular localization, and even reprogram them to create new tools for studying cell biology and treating diseases.”