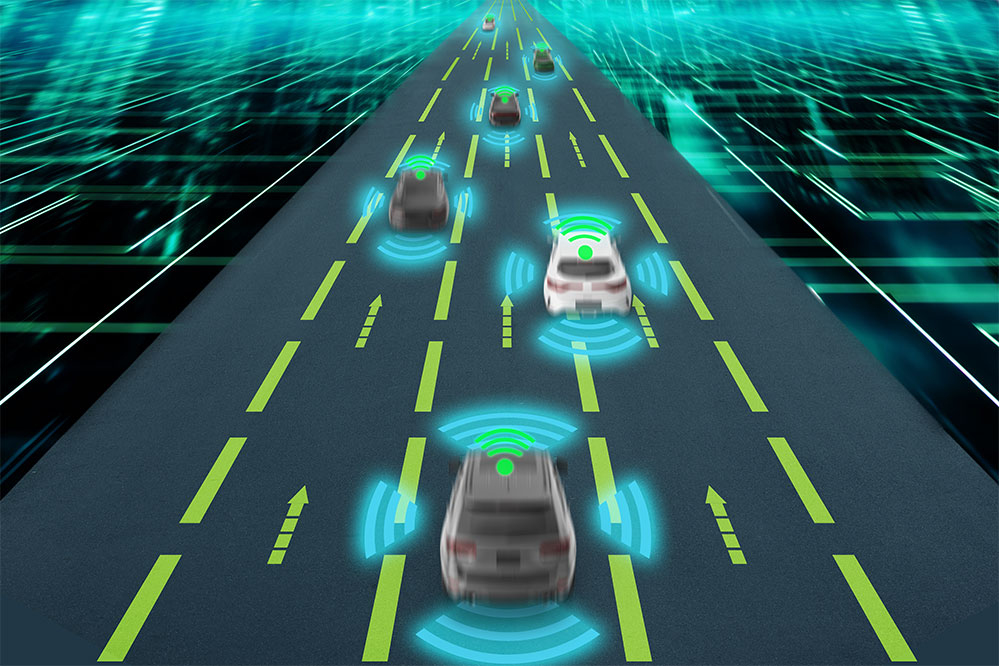
If there’s one thing that characterizes driving in any major city, it’s the constant stop-and-go as traffic lights change and as cars and trucks merge and separate and turn and park. This constant stopping and starting is extremely inefficient, driving up the amount of pollution, including greenhouse gases, that gets emitted per mile of driving.
One approach to counter this is known as eco-driving, which can be installed as a control system in autonomous vehicles to improve their efficiency.
How much of a difference could that make? Would the impact of such systems in reducing emissions be worth the investment in the technology? Addressing such questions is one of a broad category of optimization problems that have been difficult for researchers to address, and it has been difficult to test the solutions they come up with. These are problems that involve many different agents, such as the many different kinds of vehicles in a city, and different factors that influence their emissions, including speed, weather, road conditions, and traffic light timing.
“We got interested a few years ago in the question: Is there something that automated vehicles could do here in terms of mitigating emissions?” says Cathy Wu, the Thomas D. and Virginia W. Cabot Career Development Associate Professor in the Department of Civil and Environmental Engineering and the Institute for Data, Systems, and Society (IDSS) at MIT, and a principal investigator in the Laboratory for Information and Decision Systems. “Is it a drop in the bucket, or is it something to think about?,” she wondered.
To address such a question involving so many components, the first requirement is to gather all available data about the system, from many sources. One is the layout of the network’s topology, Wu says, in this case a map of all the intersections in each city. Then there are U.S. Geological Survey data showing the elevations, to determine the grade of the roads. There are also data on temperature and humidity, data on the mix of vehicle types and ages, and on the mix of fuel types.
Eco-driving involves making small adjustments to minimize unnecessary fuel consumption. For example, as cars approach a traffic light that has turned red, “there’s no point in me driving as fast as possible to the red light,” she says. By just coasting, “I am not burning gas or electricity in the meantime.” If one car, such as an automated vehicle, slows down at the approach to an intersection, then the conventional, non-automated cars behind it will also be forced to slow down, so the impact of such efficient driving can extend far beyond just the car that is doing it.
That’s the basic idea behind eco-driving, Wu says. But to figure out the impact of such measures, “these are challenging optimization problems” involving many different factors and parameters, “so there is a wave of interest right now in how to solve hard control problems using AI.”
The new benchmark system that Wu and her collaborators developed based on urban eco-driving, which they call “IntersectionZoo,” is intended to help address part of that need. The benchmark was described in detail in a paper presented at the 2025 International Conference on Learning Representation in Singapore.
Looking at approaches that have been used to address such complex problems, Wu says an important category of methods is multi-agent deep reinforcement learning (DRL), but a lack of adequate standard benchmarks to evaluate the results of such methods has hampered progress in the field.
The new benchmark is intended to address an important issue that Wu and her team identified two years ago, which is that with most existing deep reinforcement learning algorithms, when trained for one specific situation (e.g., one particular intersection), the result does not remain relevant when even small modifications are made, such as adding a bike lane or changing the timing of a traffic light, even when they are allowed to train for the modified scenario.
In fact, Wu points out, this problem of non-generalizability “is not unique to traffic,” she says. “It goes back down all the way to canonical tasks that the community uses to evaluate progress in algorithm design.” But because most such canonical tasks do not involve making modifications, “it’s hard to know if your algorithm is making progress on this kind of robustness issue, if we don’t evaluate for that.”
While there are many benchmarks that are currently used to evaluate algorithmic progress in DRL, she says, “this eco-driving problem features a rich set of characteristics that are important in solving real-world problems, especially from the generalizability point of view, and that no other benchmark satisfies.” This is why the 1 million data-driven traffic scenarios in IntersectionZoo uniquely position it to advance the progress in DRL generalizability. As a result, “this benchmark adds to the richness of ways to evaluate deep RL algorithms and progress.”
And as for the initial question about city traffic, one focus of ongoing work will be applying this newly developed benchmarking tool to address the particular case of how much impact on emissions would come from implementing eco-driving in automated vehicles in a city, depending on what percentage of such vehicles are actually deployed.
But Wu adds that “rather than making something that can deploy eco-driving at a city scale, the main goal of this study is to support the development of general-purpose deep reinforcement learning algorithms, that can be applied to this application, but also to all these other applications — autonomous driving, video games, security problems, robotics problems, warehousing, classical control problems.”
Wu adds that “the project’s goal is to provide this as a tool for researchers, that’s openly available.” IntersectionZoo, and the documentation on how to use it, are freely available at GitHub.
Wu is joined on the paper by lead authors Vindula Jayawardana, a graduate student in MIT’s Department of Electrical Engineering and Computer Science (EECS); Baptiste Freydt, a graduate student from ETH Zurich; and co-authors Ao Qu, a graduate student in transportation; Cameron Hickert, an IDSS graduate student; and Zhongxia Yan PhD ’24.